Why are AI-powered writing products so similar on the market? How can they be different?
- Bayes Tang
- 7 days ago
- 3 min read
Updated: 2 days ago
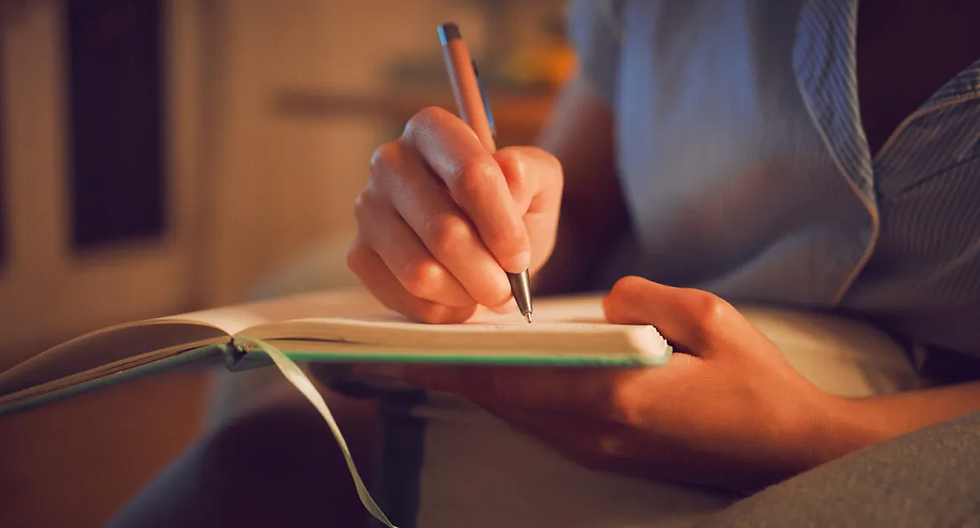
Lately, I’ve noticed that AI-powered writing and note-taking tools are becoming increasingly similar. At first, this seemed like a coincidence, but after some thought, I realized it’s both expected and concerning. It’s a sign of industry growth but also a lack of true innovation. I want to share my thoughts and hear what you think.
I'm responsible for product design & development of remio, an innovative and unique AI knowledge base and note-taking software for global users. Market and competitor research is an integral part of our work, so my perspective may not be entirely objective. If you are interested, please visit: https://www.remio.ai/waitlist to join the beta test. Of course, as a user and product enthusiast, I'm offering both objective and professional insights.
In my view, most AI-powered writing products and note-taking tools today fall into 3 categories:
Pre-AI Era Tools (e.g., Evernote, Notion, Obsidian)
These aren’t heavily affected by the AI wave yet. Each follows a unique path—Notion, for example, is focusing more on low-code and collaboration.
AI Writing Tools (e.g., Copy.ai, Frase, QuillBot)
AI Note-Taking Tools (e.g., Fathom, tl;dv, Granola)
The last 2 categories—AI writing and AI note-taking—are where the real problem lies. These products are becoming highly similar in how they work and what they offer. Why?
Beyond fundamental factors like rushing to gain first-mover advantage and cost control, there are some unavoidable reasons:
Same Core Technology: Since the emergence of ChatGPT, everyone recognizes the breakthroughs in AI capabilities. Many products have been built on similar AI models, mainly focusing on text generation.
Similar User Needs: People naturally seek convenience; unless required by their profession, they are unlikely to engage in writing or note-taking. The most widespread necessary scenarios involve producing reports, research, and academic papers, giving rise to the AI Content Generation category. Another common scenario is meeting minutes, which, when combined with speech-to-text capabilities, form the AI note-taking category. It’s no exaggeration to say that currently, among AI note-taking products, at least seven focus on meeting scenarios.
Shared Data Sources: AI products heavily rely on publicly validated data, AKA “world knowledge.” These datasets are trained on public data, lacking personalization, resulting in generated content with similar structures, convergent styles, and a lack of individuality — what is often referred to as a strong “looks like AI.”
Many companies follow a safe, fast approach:
Pick a very clear target audience.
Use existing AI models and data.
Focus on the best return on investment (ROI).
This creates polished but generic products. In the long run, I don’t think this is sustainable for most businesses.
So, the question becomes: can they be different? I believe it’s difficult but possible, with some strategies:
Focus on the Process, Not Just the Result
As mentioned, there are few scenarios where users need to produce text. However, even in information and knowledge management, users act more as consumers than producers. Why not focus on the process of users managing information?
Be Patient
Don’t rush; the ultimate winners will be applications with innovative applications and user experiences. This requires time to refine, determination, and perhaps even a stroke of luck.
Make It Personal
Personalization is key. World knowledge is undoubtedly valuable and serves as a good supplement for users. However, the data closest to production is in the hands of users. Ensuring data security, it’s essential to build differentiated data assets.
What do you think? Have you noticed this trend too? Let’s discuss!
Comments